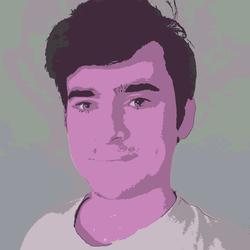
raML - Back at it!
Progress Couple of days later
Finally got to implementing mini batches to enable fast training. Was observing very strange behavior, only later realized I forgot to change Sigmoid to Identity activation in the output layer, so no wonder the model didn't exactly give good predictions for housing prices :P
Anyway, after fixing some things, here is a mini batch training of the mnist dataset ("but Ramil, it doesn't make sense
to use MSE here", yes, yes, but I don't have Softmax implemented correctly yet). So this is the fitting going once through all data with 32 sized batches. The good thing is that it seems noisy, as it should be.
Reminder to future me: Next major goal to speed things up is to write convolutions.